Machine learning software offers a proactive path to water infrastructure resilience
- Greg Johnston
- Aug 8, 2024
- 5 min read
Updated: Oct 29, 2024
By Greg Johnston

In March, a sewage pipe failure led to 135 million litres of untreated sewage spilling to Winnipeg’s Red River. In June, a catastrophic water main break in Calgary led to a state of emergency and a month of water usage restrictions. Machine learning (ML) and advanced analytics software can help cities prevent such situations.
Citing Canada’s Infrastructure Report Card 2019, Tricia Stadnyk, a Professor in Civil Engineering at the University of Calgary’s Schulich School of Engineering and Canada Research Chair in Hydrological Modelling, has repeatedly stressed that about 30 per cent of the water infrastructure in Canada is at or near the end of its service life.
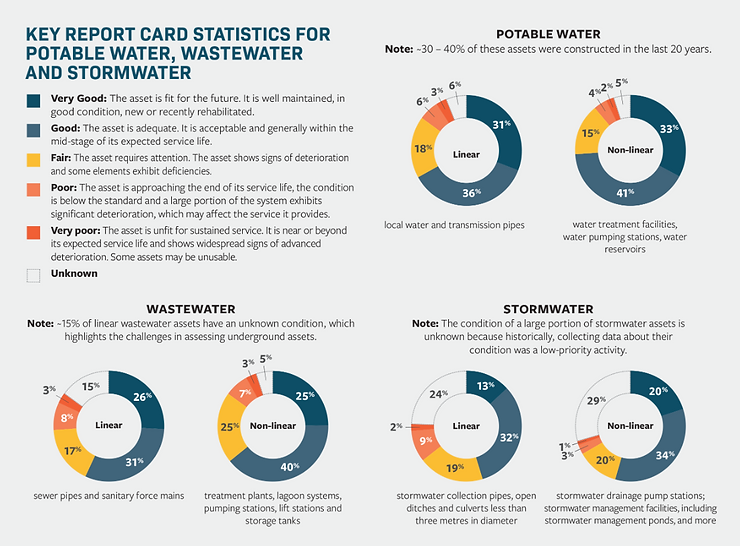
Professor Stadnyk stated that “We have to remember that underneath these paved roads and soils is a whole network of pipes that we can’t see. And so unlike buildings that are aging that are aging and starting to crumble … engineers can’t see the cracks and say ‘You know what, maybe we should do some repair work’. The infrastructure that’s under our feet … is hidden under the soil, and it is very labour intensive to be able expose it to actually do an examination.”
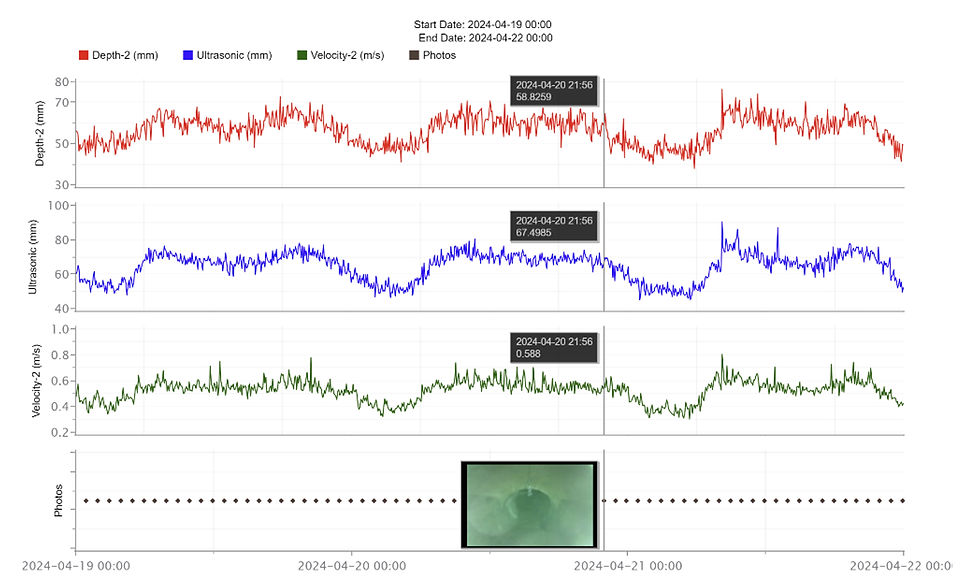
Monitoring critical water infrastructure with a single time-series data management system that solves the common problem of consolidating disparate data sources while enacting labour-saving automation is a worthwhile and achievable goal for water system monitoring programs.
Make predictions on where problems are likely to occur
Photo data channels can store years of images for comparison purposes. Adding a camera data channel allows viewing of camera images alongside level and velocity data to gauge the operational efficiency of the site over time
Identify and track cracks and structural damage through Inflow and Infiltration (I&I) analysis from sensor data then prioritize maintenance as required
Investigate unusual site activity. Set alarms based on incoming sensor data. Use a photo data channel to easily identify the source of an infrastructure issue
Identify sensor anomalies and problems using machine learning algorithms. A confirmation of bad sensor data can assist in making the decision to replace monitoring equipment before false readings lead to false assumptions about the true state of infrastructure being monitored
Run models using incoming sensor data using machine learning or other advanced statistical methods to make predictions on where a problem is likely to occur. Compare your mechanical model data with your statistical model and incoming sensor data to better understand how your system is operating
Time-series data monitoring significantly enhances the ability of water infrastructure operators to proactively create priority maps for future maintenance work, predict problem areas and in so doing reduce the time and associated expense required for data analysis. Here are four examples:
350 sensors and 30 rain gauges capture over a billion points of data
In the Region of Peel, which provides water and wastewater services to 1.5 million residents and over 175,000 businesses, a multi-year Flow and Rainfall Data Delivery project initiated by infinitii ai’s engineering integration partner SCG Flowmetrix is installing 350 sensors throughout the sewer network and more than 30 rain gauges to monitor and collect data for hydraulic modelling, capacity assessments, inflow and infiltration remediation, flood forecasting, regulatory reporting requirements, and more.
Real-time data provides critical and actionable information during extreme weather events and will enable proactive sewer network maintenance. Streaming analytics allows users to view custom data transformations from production ready models, including predictions.
“Over one billion points of data will be captured and analyzed over the life of this project, and advanced machine-learning and quality assurance software will drive more actionable insights for Peel Region,” stated Sam Mills, Vice President at SCG Flowmetrix.
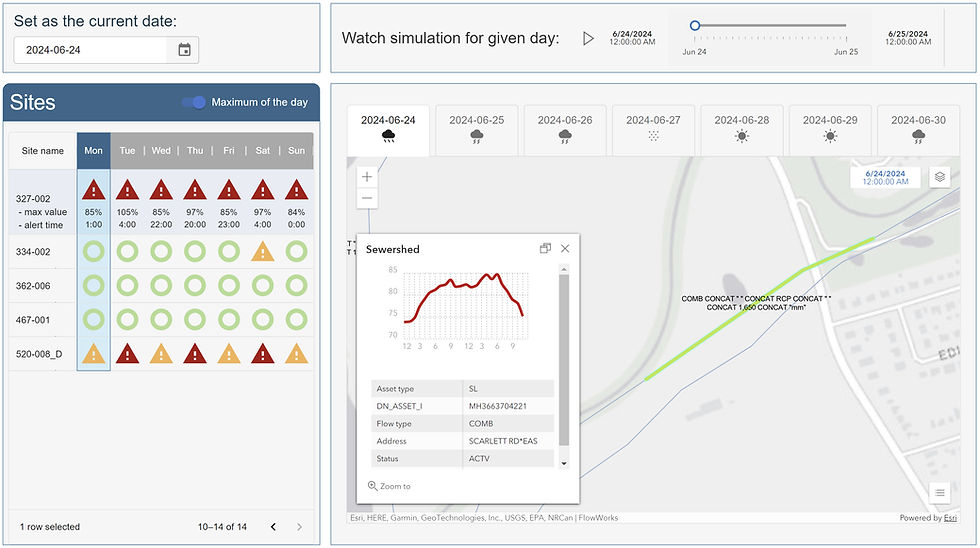
AWWA Innovation Award for machine learning
The Regional Municipality of York (York Region) earned an American Water Works Association (AWWA) Innovation Award for developing a machine learning project for managing I&I. York Region designed a machine learning model as part of its ongoing I&I Reduction Strategy.
The model uses machine learning to process raw data into actionable information to help make proactive and better-informed decisions to manage infrastructure. Real-time and historical data are integrated to create a priority map that informs future operations and maintenance work. It can predict the way the system would respond to hypothetical precipitation events.
78% reduction in analysis time amounts to $40,000 per analysis in savings
York Region has realized a 78% reduction in analysis time when compared to the two weeks it took to do the analysis manually. This amounts to approximately $40,000 in labor costs per analysis of all nine of the municipalities within the region.
The engineers at Vancouver-based Kerr Wood Leidal Associates Ltd. deploy custom data transformations with the infinitii face pro application for streaming analytics on behalf of its clients to manage and operationalize production-ready models that generate new data channels such as predictions. They build models from sensor data or other data sources using existing scripts created in Python.
Build models from sensor data using existing Python scripts
“What we love about infinitii face pro is that our data engineers and scientists can write Python and R scripts using their preferred development environment and then copy and paste the code into face pro and have it running system wide quickly and efficiently,” said Luis Galindo, Senior Data Scientist at Kerr Wood Leidal Associates Ltd. “This provides better data-driven insights for making faster, more informed decisions, particularly with real-time and forecasting applications.”
The types of advanced calculations now easily performed by KWL’s engineering team include Soil and Water Integrated Model (SWIM) calculations that track and predict climate and land use change impacts at a regional scale, and Evapotranspiration (ET) calculations used to estimate soil-moisture storage based on precipitation deficit and the maximum water-holding capacity of the soil.
QA/QC on sensor data outliers that trigger false alerts
The City of Montreal has undertaken a project to perform Quality Assurance and Quality Control (QA/QC) on any sensor data outliers that may distort results or trigger false alerts. Machine Learning models provide insight on data identified from sensor anomalies. Problem data is identified for further action in the following ways:
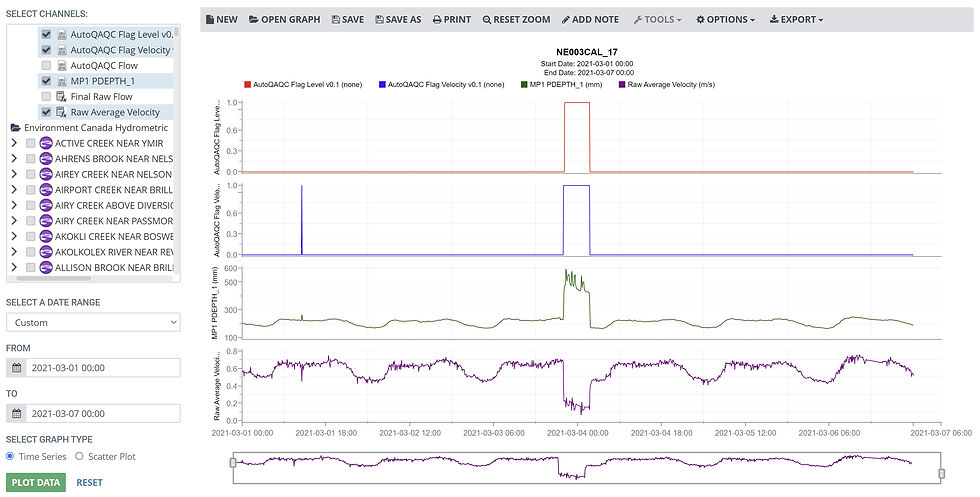
Data anomalies are flagged for further investigation by engineers and data analysts in their manual workflow
Certain anomalies may be deemed acceptable, and automatically eliminated from the data stream
Other anomalies may be automatically substituted with predicted data values to limit impact on real-time predictions

All of these innovative machine learning applications are in keeping with AWWA’s Water 2050 Think Tank Report that recommends machine learning (ML) and artificial intelligence (AI) solutions. AWWA recommendations include:
Employ digital solutions such as AI and ML to optimize efficiency, operations and water quality
Apply real-time monitoring, predictive analytics and material science to create 'eternal infrastructure' and support resilient resources
Why wait until 2050 for the next catastrophic infrastructure failure? The time is now for water infrastructure professionals to undertake AI and ML initiatives that can significantly advance water infrastructure resilience.
Comments